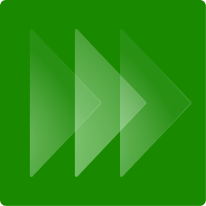
Get started with Bodo
Get started with Bodo by setting up your local environment and running simple example programs.
Access all your Bodo resources in one place
If you are new to Bodo, this section has a couple of quick start guides to help you get started with Bodo.
This section provides a collection of guides to help you go from beginner to expert with Bodo. Choose a guide from the list below to get started.
This section provides guides to help you install and set up Bodo on your local machine or get onboarded on Bodo Platform on AWS or Azure.